Healthcare is generally considered to have been slower to deploy artificial intelligence and machine learning solutions than industries like manufacturing and e-commerce, with frequently cited reasons for this lag in adoption including the potential risk to patient safety and the complexity of medical imaging, diagnosis, and research. But over the last five years, we’ve increasingly seen artificial intelligence, and especially machine learning, gaining traction with medical professionals in hospitals, clinics, and research labs across the world.
The accelerated deployment of artificial intelligence and machine learning in medicine and healthcare shows no signs of stopping. In 2021, Grand View Research valued the global market for artificial intelligence in healthcare at $10.4 billion and expects this market to grow at a 38.4% compound annual growth rate (CAGR) over the next decade, over three times the rate at which Apple has grown in the last ten years. Apple’s ten-year revenue CAGR.
But how exactly are AI technologies changing the face of healthcare, and how might you contribute to this change? In this article, we’ll explore the impact machine learning is having on patient diagnosis, treatment, and engagement, as well as healthcare administration and drug discovery — and provide some tips for how you might get involved in the future of medicine.
Precision Medicine
One of the greatest impacts machine learning is already having in healthcare is in precision medicine, the practice of predicting which treatments will work for a patient given their specific background and physiological characteristics. Indeed, in Future Healthcare Journal, an international journal published by the Royal College of Physicians, Thomas Davenport and Ravi Kalakota cite precision medicine as the most prevalent use of “traditional” machine learning.
As an example, Davenport and Kalakota cite a 2018 paper published in Nature Communications that reports the successful use of a machine learning model to determine drug sensitivity to popular chemotherapy treatments based on cancer patients’ unique genetic make-ups. When considered together with other patient information, including health history and symptoms, the authors of Precision Medicine, AI, and the Future of Personalized Health Care note in Clinical and Translational Science, ML-derived data on drug sensitivity promises to improve a doctor’s ability to personalize both diagnosis and prognosis.
Medical Imaging
Also supporting diagnosis and prognosis are the continually improving capabilities of deep learning, a more advanced subdiscipline of machine learning that leverages artificial neural networks. When combined with computer vision technologies, deep learning can identify diseases like cancer and even predict patient outcomes from medical images that remain inscrutable to humans.
While the extraction of diagnostic and prognostic data from details in medical images — the foundation of what is now called radiomics — isn’t new, in recent years machine learning and computer vision have teamed up to draw findings from features of visual data that the human eye would be unable to discern. According to a review of relevant research in Translational Cancer Research, these capabilities will likely only increase in the coming years, provided that there is easily accessible training data to expand the kinds of cancer that can be detected.
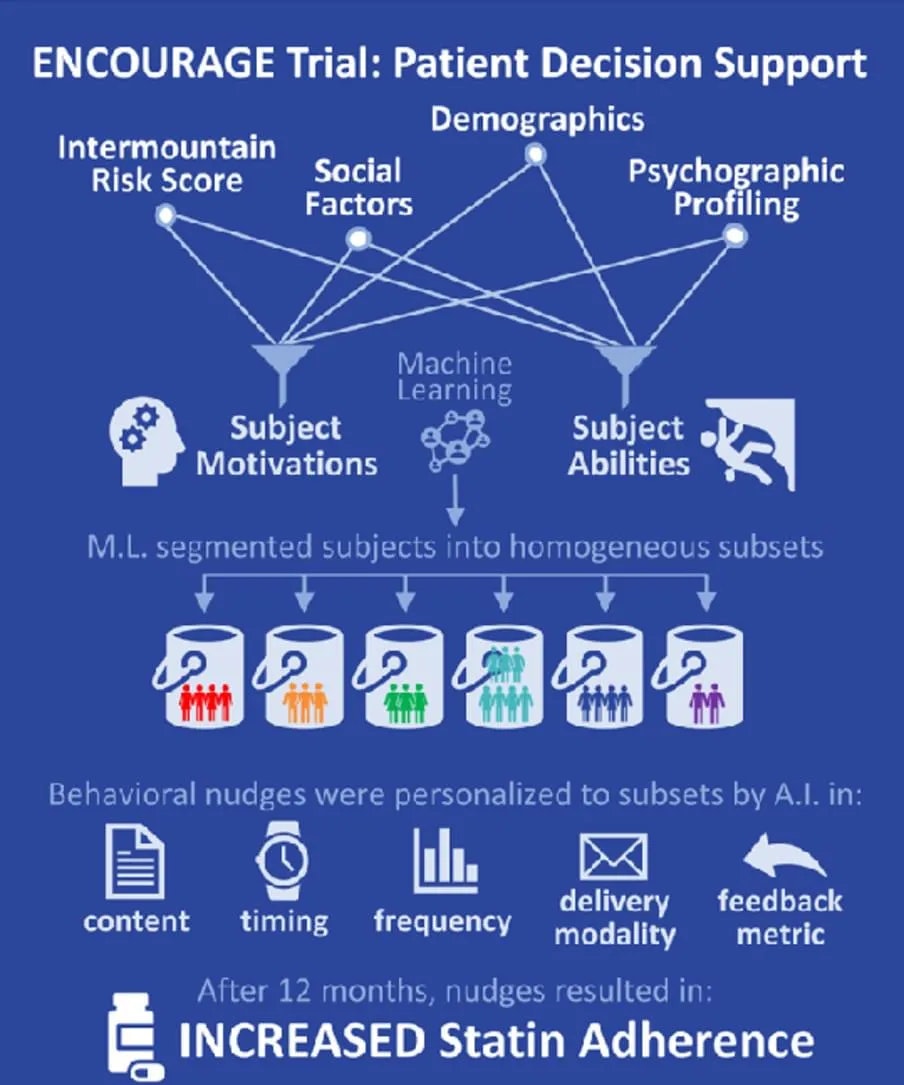
While less flashy than applications in precision medicine and medical imaging, machine learning is also playing an important role in patient engagement and adherence. Doctors can only do so much — with most health care and treatment taking place outside of the clinic — but machine learning is increasingly helping patients help themselves. Using machine learning algorithms to manage patient communications like appointment and prescription reminders and nudges to remain compliant with treatments, healthcare professionals are quickly learning how to expand their influence.
So far, these kinds of applications of machine learning have found quantifiable success. The ENCOURAGE clinical trial, which tested the efficacy of AI-driven nudges in improving adherence, found that this mode of patient communication increased statin compliance among cardiology patients in meaningful ways. While technological tools such as these might not seem cutting-edge, they have an outsized influence on public health, increasing the potential for major-event prevention and thus decreasing stress on the health system as a whole.
Healthcare Administration
Machine learning is also increasingly providing solutions for healthcare administration. Davenport and Kalakota note the ability of natural language processing (NLP) to drive efficiency in patient-provider communications and machine learning-driven data mining to streamline the insurance claim process.
Another area of healthcare administration where machine learning will increasingly make a difference is electronic health records. Harvard Business Review expects AI advancements to the efficiency and usability of electronic health record systems to accelerate in the coming years, with improvements taking the form of more sophisticated diagnostic and prognostic machine learning models, better clinical documentation assistance, and more dynamic and personalized clinical decision support that will streamline clinical workflow.
HBR also points to the potential impact of the data-captured Internet of Things (IoT) wearables in building out more comprehensive records of an individual’s health. Among the technologies they preview are blood pressure monitors and even smart T-shirts that can capture health data and seamlessly transmit it to clinicians and other healthcare professionals.
Drug Discovery
Another area of huge potential for machine learning in medicine comes in the advancements machine learning methods can bring to drug discovery. A review of its relevant applications in this sector, published under the umbrella of Nature, notes in particular machine learning’s efficacy in “target validation, identification of prognostic biomarkers and analysis of pathology data in clinical trials.” In other words, machine learning can help researchers assess whether a drug can provide a safe and therapeutic effect on its target, pinpoint useful metrics to predict future clinical events, and analyze a drug’s impact on a patient’s fluids and tissues during clinical trials.
Recently, researchers at MIT have had particular success using machine learning for target validation. Using a deep learning model called DeepBAR, these scientists have been able to accurately determine the binding potential between drug candidates and their targets — binding being essential, of course, for any possible drug efficacy.
DeepBAR offers a glimpse of an AI-driven future, but the Nature reviewers warn that while machine learning can provide support at all stages of drug discovery, it’s not a silver bullet. To be effective, machine learning algorithms need to be designed based on specific research questions and have ample amounts of high-quality medical data to train on and analyze. The difficulty of producing interpretable, repeatable results is also reportedly holding back more widespread machine learning application.
How can you get involved?
As healthcare companies continue to integrate existing artificial intelligence and machine learning capabilities and research and develop new applications, demand for qualified technologists, especially machine learning engineers, will only grow. Those that meet the call will be well-compensated: Salary.com estimates the average annual machine learning engineer salary in the US to be $123,134, far higher than the average annual salary in the US, which the U.S. Bureau of Labor Statistics estimated to be $58,260 in 2021.
If the prospect of getting paid handsomely to deploy new kinds of artificial intelligence and machine learning in medicine and healthcare interests you, there are several ways you can get involved, depending on your previous experience.
For those still in high school and interested in designing machine learning solutions for healthcare, the best career path likely starts with a bachelor’s degree program in computer science, artificial intelligence, or machine learning, potentially paired with an additional major or a minor in biology or a healthcare-related field. Head over to our programs section to see our recommendations for great computer science, artificial intelligence, and machine learning bachelor’s programs.
For those with previous medical experience looking to shift careers, you might instead consider a master’s or Ph.D. program in artificial intelligence or machine learning. Either will allow you to complement your existing expertise with important computer science skills, with a Ph.D. program in particular offering opportunities to contribute to new and exciting research initiatives. Certain of these programs can be taken part-time and/or remotely, allowing you to continue working as you study.
If you’re a healthcare administrator looking to learn more about how AI and ML solutions might be deployed at your clinic or hospital, there’s no need to go back to school for one of these longer-term degree programs. Our short-course recommendation page features strong options focusing specifically on how machine learning can be deployed in a healthcare setting to drive efficiency and patient outcome.
After you pick a program that’s right for you, head over to our applications page for the latest tips and tricks that will help you stand out from the crowd of applicants and make the next step on your AI journey. Forward!