Regardless of size, regardless of industry: machine learning is helping businesses increase efficiency, boost productivity, and better prepare for the future. According to an MIT Technology Review survey of over 300 top businesses, 60% were already employing machine learning solutions in 2017, a number that has only grown in the years since.
For these companies, the investment in machine learning has already reaped rewards. Deloitte reports that businesses reported between 200 and 500% return on their machine learning investments — an upside that any C-suite would have a hard time turning down. It’s no surprise that demand for machine learning solutions is growing at a stunning rate: by 2028, Grand View Research projects the global AI market to expand by over 40%, with revenues forecasted to top $900 trillion worldwide.
While the numbers are shocking, they don’t tell the whole story. What’s the impact of machine learning in business once implemented? What do these solutions look like, and what problems are they solving? In this article, we’ll dive into the ways machine learning is making a difference for corporations across the world, with use cases that will highlight the real change that machine learning is helping to bring about.
We’ll also give you some tips for how you can get involved in the future of machine learning in business, regardless of whether you are contemplating a degree, plotting out your next career move, or a seasoned exec looking to capture the efficiency and productivity benefits of machine learning for your company.
What is machine learning?
Before we go too deep into the impact of machine learning in business, it’s important to have an understanding of what machine learning is.
A subset of artificial intelligence, machine learning focuses on the development of mathematical algorithms that allow computers to progressively improve their capabilities — “learning” as they go. As Stuart Russell and Peter Norvig put it in Artificial Intelligence: A Modern Approach, the leading AI textbook, in machine learning “a computer observes some data, builds a model based on the data, and uses the model as both a hypothesis about the world and a piece of software that can solve problems.”
There are three fundamental paradigms of machine learning, all of which have business applications: supervised learning, unsupervised learning, and reinforcement learning. You’ll frequently also hear about another subset of machine learning, deep learning, which also has substantial applications for businesses.
Supervised Learning
Supervised learning refers to the use of labeled data sets — where each piece of data is tagged and classified — to train a machine learning algorithm to give the correct output when fed an input. Essentially, supervised learning helps algorithms build an ML model by learning by example.
A common use of supervised learning is consumer sentiment analysis, with machine learning algorithms analyzing vast amounts of data from sources like social media to better understand customer emotions, intent, and actions.
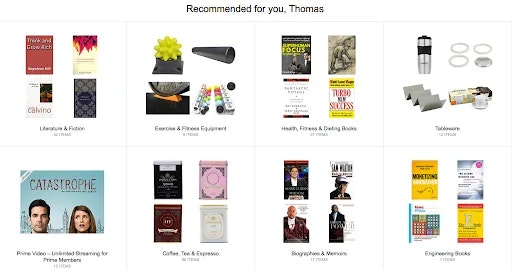
Image Credit: www.rejoiner.com
Unsupervised Learning
Unsupervised learning refers to the use of unlabeled data sets to train a machine learning algorithm. Rather than learning by example, unsupervised ML algorithms are written to be able to make sense of the data themselves: discovering patterns and relationships, forming clusters, and cutting through the data to get to the most important data points.
In business, unsupervised learning can be used to create product recommendations for e-consumers or even develop customer personas based on buying habits and internet activity.
Reinforcement Learning
Reinforcement learning differs from supervised and unsupervised learning in that it is explicitly goal-oriented, with machine learning algorithms written to behave in ways that will maximize a numerical reward as they engage with a complex environment, usually through things called Markov decision processes.
Reinforcement learning is applied throughout the industry, including in teaching cars how to drive themselves.

Image Credit: www.CNET.com
Deep Learning
While not one of these key paradigms, another important method of machine learning is deep learning, where “artificial neural networks” (ANNs) of algorithms inspired by the synapses and neurons of the human brain are used for tasks in natural language processing, computer vision, and many other areas.
One notable application of deep learning in business comes on the assembly line, where deep learning models can learn to predict when robots and machines will require maintenance to delay costly breakdowns and delays.
With supervised learning, unsupervised learning, and reinforcement learning we saw some basic examples of how machine learning is driving businesses forward — but there are several other ways it is doing so, including:
Decision Support
Machine learning provides crucial support to business execs by identifying and analyzing data to develop insights that can drive decision-making. When discussing data-driven decision-making, you’ll often hear data analysts, data scientists, machine learning engineers, and corporate executives use the terms business intelligence and business analytics. Though some will use these terms interchangeably, as Harvard Business School notes, they have key differences that are important to keep in mind.
Business Intelligence
According to Oracle, business intelligence analyzes “historical and current data to understand what happened in the past and what is happening now,” an analysis that can help streamline and improve day-to-day business operations. In this, machine learning can play a prominent role. Through unsupervised learning, for example, algorithms can discover patterns and anomalies that can provide insights into employee or technological inefficiencies, the first step to solving them.
Business Analytics
Business analytics differs from business intelligence in being more forward-looking. As Oracle puts it, “business analytics builds on the foundation of business intelligence and attempts to make educated predictions about what might happen in the future.” Whereas in business intelligence, unsupervised learning might be employed for its ability to recognize patterns and anomalies, in business analytics supervised learning might be used to build predictive models to give decision-makers visibility into not just today’s markets, but tomorrow’s.
Intelligent Automation
In intelligent automation, machine learning teams up with other AI subdisciplines like computer vision and natural language processing to execute complex and time-consuming tasks more quickly, effectively, and inexpensively than a human could. This might come in the form of streamlining supply-chain management or quickly processing enormous tranches of documents.
Customer Support
Machine learning is also frequently deployed to improve how a business engages with its customers. A familiar application of machine learning that millions of consumers engage with daily comes in the form of chatbots that free up both manpower and capital as many e-retailers’ first lines of customer service. And as we mentioned above, machine learning also provides customer support — and provides businesses with a powerful value proposition — by driving the personalized product and content recommendations now ubiquitous in our online experiences.

Image Credit: www.mygreatlearning.com
Machine learning additionally provides solutions for businesses for whom fraud detection is critical, like banks and credit card companies. As fraud-detecting outfit Ravelin notes, machine learning allows the once resource-intensive task of fraud detection to be performed more quickly, accurately, scalably, and efficiently than traditional methods by training a supervised learning model off of a business's data.
As businesses continue to integrate existing machine learning capabilities and researchers continue to develop new applications, demand for qualified technologists, especially machine learning engineers, will only grow. This rising demand will mean lucrative salaries: Salary.com estimates the average annual machine learning engineer salary in the US to be $123,134, more than double the average annual salary in the US, which the U.S. Bureau of Labor Statistics pegged at $58,260 in 2021. The same holds for data scientists, a profession that utilizes many of the same machine learning skills while focusing more squarely on the data pipeline and business insights. Currently, Salary.com estimates the range for an average annual data scientist salary in the US to be $123,276 to $152,489.
If the prospect of getting paid handsomely to deploy new machine learning models interests you, there are several ways you can get involved, depending on your previous experience.
For those still in high school, the best career path likely starts with a bachelor’s degree program in computer science, artificial intelligence, or machine learning, potentially paired with an additional major or a minor in economics, business, or even supply chain management. Head over to our programs section to see our recommendations for great computer science, artificial intelligence, and machine learning bachelor’s programs.
For those with previous business experience looking to shift careers, you might instead consider a master’s or Ph.D. program in artificial intelligence or machine learning. Either will allow you to complement your existing expertise with important computer science skills, with a Ph.D. program in particular offering opportunities to contribute to new and exciting research initiatives. Certain of these programs can be taken part-time and/or remotely, allowing you to continue working as you study.
If you’re a business executive looking to learn more about how AI and ML solutions might be deployed at your company, there’s no need to return to school for longer-term degree programs. Short courses like MIT’s Machine Learning in Business can immediately help begin implementing machine learning solutions at your company. Our short course recommendation page features strong options focusing specifically on how machine learning can be deployed in a business setting to drive efficiency, productivity, and, ultimately, the bottom line.
After you pick a program that’s right for you, head over to our applications page for the latest tips and tricks that will help you stand out from the crowd of applicants and make the next step on your AI journey. And if you’re interested in how machine learning is making a difference in other industries, check out our overview of machine learning applications or our article on machine learning in healthcare. Forward!