The Basics of Solar Energy
Understanding the basics of solar energy is fundamental to appreciating its transformative potential in addressing our global energy needs. In this section, we will delve into the intricacies of photovoltaic cells, explore current challenges in solar energy harvesting, and emphasize the imperative for advancements in efficiency and optimization.
Explanation of AI applications in energy
Solar energy, at its core, revolves around the ingenious functionality of photovoltaic (PV) cells. These cells are the building blocks of solar panels, responsible for converting sunlight into electricity. Here's a closer look at their fascinating operation:
Photovoltaic Phenomenon: PV cells operate on the principle of the photovoltaic effect. When sunlight, composed of photons, strikes the semiconductor material within the cell, it induces a release of electrons. This flow of electrons creates an electric current, initiating the generation of electrical power
Composition of PV Cells: Most commonly, these cells are made from crystalline silicon, a material known for its semiconductor properties. Thin-film technologies and emerging materials like perovskite are also gaining traction, offering alternative paths to enhance efficiency.
Solar Panels and Arrays: PV cells are arranged in solar panels, and these panels can be further combined into larger arrays to generate substantial amounts of electricity. The arrangement and connection of these cells play a crucial role in the overall efficiency of the solar energy system.
Current Challenges in Solar Energy Harvesting
While the promise of solar energy is immense, the industry faces certain challenges that hinder its widespread adoption and efficiency. Understanding these challenges is vital for devising effective solutions:
Intermittency and Weather Dependency
Solar energy production is highly dependent on sunlight, making it intermittent and weather-sensitive. Cloudy days and nighttime halt electricity generation, necessitating backup solutions for continuous power supply
Energy Storage Limitations
The ability to store excess energy for use during non-productive periods is crucial. Current battery technologies have limitations in terms of capacity, efficiency, and cost, impacting the seamless integration of solar power into the grid.
Land Use and Aesthetic Concerns
The extensive land requirements for solar farms raise concerns about land use and its impact on ecosystems. Additionally, aesthetic considerations and public perception can be hurdles in deploying solar installations in urban or natural landscapes.
Overview of How AI Works with Solar Energy Systems
Artificial Intelligence (AI) applications in the energy sector have ushered in a new era of efficiency, sustainability, and innovation. Here's how the synergy between these two fields unfolds:
Predictive Analytics
AI excels at predictive modeling. In solar energy systems, AI algorithms analyze historical and real-time data to predict energy production, considering factors like sunlight intensity, weather conditions, and even the angle of solar panels. This enables more accurate forecasting and grid management.
Benefits:
Proactively addressing issues prevents unexpected system failures.
Timely maintenance ensures that components operate within optimal conditions, prolonging their lifespan.
Example: An AI system monitors the performance of solar panels and predicts when specific panels may require cleaning or maintenance based on historical data related to degradation patterns.
Dynamic System Optimization
Solar energy systems are inherently dynamic, with energy production affected by varying environmental conditions. AI adapts to these changes in real-time, optimizing the system's configuration for maximum efficiency.
Benefits:
AI identifies issues as they arise, preventing prolonged periods of suboptimal performance.
Some AI systems can autonomously implement corrective measures, reducing the need for manual intervention.
Example: An AI algorithm detects a drop in energy production from a specific set of panels, signaling a potential fault. The system then redirects energy production to other panels while alerting maintenance for further investigation.
Fault Detection and Maintenance
AI is instrumental in identifying anomalies or faults in the solar energy system. Through continuous monitoring, it can detect issues such as faulty panels or suboptimal performance.
Benefits:
AI-enhanced weather predictions enhance the accuracy of solar output forecasts.
The system adjusts its configuration based on anticipated weather conditions, ensuring efficient energy production.
Example: An AI-driven solar energy system integrates weather data to anticipate an upcoming cloudy period. In response, it adjusts the orientation of solar panels to capture maximum sunlight during clearer intervals, optimizing energy production.
Getting Started with AI in Solar
As we move toward more sustainable energy solutions, using AI in solar projects has become crucial. It helps improve efficiency, predict maintenance needs, and unlock the full potential of solar infrastructure.
Basics of integrating AI into existing solar setups
Incorporating Artificial Intelligence (AI) into existing solar setups is a strategic move towards unlocking the full potential of renewable energy. The process involves a thoughtful integration that aligns with the specific needs of the solar infrastructure.
Assessment of Current Infrastructure
Before venturing into AI integration, it's crucial to conduct a thorough assessment of the existing solar setup. This involves gaining insights into the specifics of solar panels, inverters, and monitoring systems currently in use.
The findings from this assessment become the bedrock for customizing AI solutions, ensuring they seamlessly complement and enhance the current infrastructure.
Data Accessibility and Compatibility
AI thrives on data, and ensuring that the solar system's data is accessible and compatible with AI algorithms is crucial. This may involve upgrading or installing sensors and data collection devices to gather real-time information about energy production, environmental conditions, and system performance.
Training and Familiarization
As AI becomes a part of the solar ecosystem, providing training and familiarization to the personnel responsible for system maintenance and operation is key. Understanding how AI algorithms function, interpret data, and make decisions empowers the team to optimize the system effectively.
Key Components Needed for AI-Enabled Solar Systems
Before delving into the intricacies of AI-driven solar energy data, it's crucial to establish clear Key Performance Indicators (KPIs) that serve as benchmarks for success. These indicators provide a quantitative measure of the system's performance and guide the evaluation of its effectiveness. Here's a detailed look at this foundational step:
Energy Output and Efficiency
The primary KPIs in solar energy systems include the amount of electricity generated and the overall efficiency of the system. These metrics provide a direct measure of the system's capability to convert sunlight into usable energy.
Predictive Accuracy
Assessing the accuracy of AI algorithms in predicting solar energy generation is paramount. KPIs related to predictive modeling, such as forecasting accuracy and deviation from actual energy production, ensure the reliability of AI-driven predictions.
Maintenance Downtime
KPIs related to system downtime due to maintenance activities are critical. Minimizing downtime ensures continuous energy production and improves the overall economic viability of the solar energy system.
Monitoring and Analyzing AI-Driven Solar Energy Data
With established KPIs in place, the next step involves the continuous monitoring and analysis of data generated by AI-driven solar energy systems. This process involves the integration of sophisticated monitoring tools and data analytics platforms:
Real-Time Monitoring
Implementing real-time monitoring systems allows for instant visibility into the performance of solar panels and associated components. AI algorithms continuously analyze data streams, providing immediate insights into energy generation patterns.
Weather and Environmental Factors
AI's capability to process vast amounts of data enables the integration of real-time weather forecasts and environmental conditions. Monitoring how these factors impact energy production allows for proactive adjustments and better decision-making.
Anomaly Detection
AI excels at identifying anomalies or deviations from expected patterns. Implementing anomaly detection algorithms helps in quickly identifying issues such as equipment malfunctions, shading, or other factors affecting energy output.
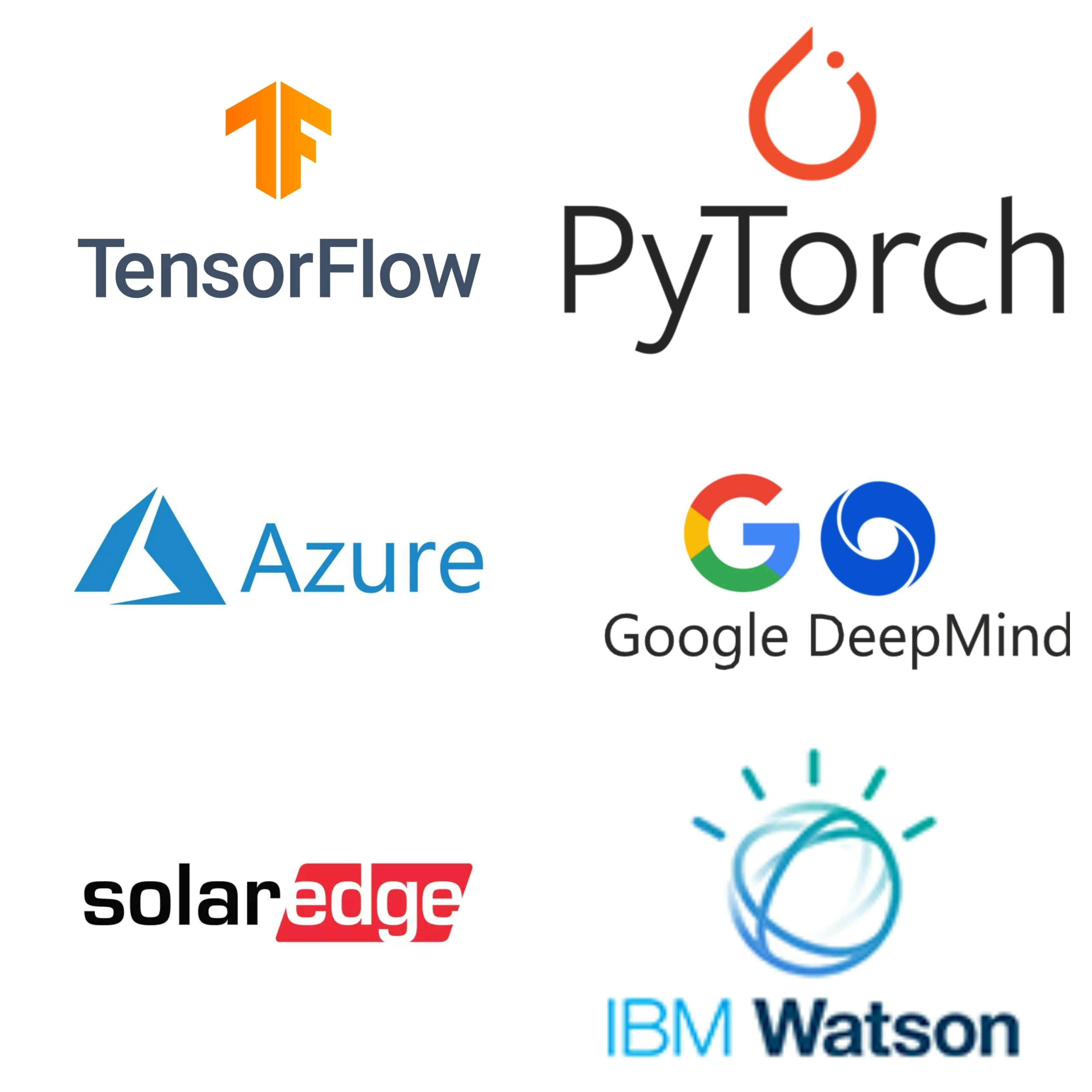
Source: AIFWD.com
Choosing the Right AI Tools for Your Solar Project
The landscape of AI tools for solar projects has expanded significantly, offering a diverse range of solutions to enhance energy efficiency and system optimization. Understanding the available tools and platforms is crucial for making informed decisions. Here's an overview of key AI tools and platforms:
TensorFlow
An open-source machine learning library developed by Google, TensorFlow is widely used for building and training machine learning models. Its versatility makes it suitable for various applications in solar energy, from predictive analytics to image recognition.
PyTorch
Another popular open-source machine learning library, PyTorch is known for its dynamic computational graph, making it particularly suitable for research and experimentation in AI applications for solar projects.
Microsoft Azure AI
Microsoft's Azure AI platform offers a comprehensive suite of AI services, including machine learning, cognitive services, and data analytics. It provides tools for building, training, and deploying AI models tailored to the specific needs of solar energy systems.
IBM Watson
IBM Watson offers AI and machine learning solutions designed to analyze large datasets and derive actionable insights. Its capabilities can be harnessed for predictive maintenance, fault detection, and optimization in solar projects.
Google's DeepMind and Solar Forecasting
In collaboration with DeepMind, Google implemented AI to improve the accuracy of solar energy forecasting for its data centers. The AI system predicted energy production 36 hours in advance with remarkable precision, aiding in efficient grid management.
SolarEdge
SolarEdge, a leading provider of solar inverters, integrates AI algorithms into its inverters to enhance energy harvesting and system performance. These inverters use machine learning to optimize the conversion of DC to AC power, maximizing overall efficiency.
PowerHub
PowerHub utilizes AI in its asset management platform for solar projects. The AI-driven system streamlines project management, analyzes performance data, and provides actionable insights to improve overall operational efficiency.
Considerations for Selecting the Right Tools for Specific Needs
Choosing the right AI tools for a solar project requires a thoughtful evaluation of specific needs, goals, and system requirements. Consider the following factors to make informed decisions:
Scalability: Ensure that the chosen AI tools can scale with the size and complexity of your solar project. Scalability is crucial for accommodating increased data volume and evolving system requirements.
Interoperability: Select tools that can seamlessly integrate with existing hardware, software, and communication protocols within your solar energy infrastructure. Interoperability facilitates smooth implementation and avoids disruptions.
Adaptability: AI tools should be adaptable to dynamic environmental conditions and changing energy demands. Look for tools with adaptive algorithms that can optimize system performance in real-time.
Ease of Use: Consider the user-friendliness of the AI tools, especially if they will be used by non-experts in machine learning. Intuitive interfaces and clear documentation contribute to efficient implementation and ongoing management.
Step-by-Step Guide for Integrating AI into Solar Energy Infrastructure
The integration of Artificial Intelligence (AI) into solar energy systems requires a systematic approach to ensure a seamless transition and optimal performance. Here's a step-by-step guide:
Define Objectives and Requirements
Clearly articulate the goals of integrating AI, whether it's for predictive maintenance, fault detection, or energy optimization. Identify the specific requirements of the solar project.
Assess Current Infrastructure
Conduct a thorough assessment of the existing solar setup, including hardware, software, and data collection methods. Identify potential areas for improvement and optimization.
Data Preparation and Integration
Prepare the existing data for AI analysis. This may involve cleaning and structuring data to make it suitable for machine learning algorithms. Integrate data collection devices and sensors if necessary.
Training Machine Learning Models
Train machine learning models using historical data to enable the system to learn patterns, predict outcomes, and make informed decisions. This step is crucial for the success of AI-driven applications.
Integration with Control Systems
Implement mechanisms for AI to interact with control systems. This includes enabling automated adjustments based on AI predictions and recommendations.
Testing and Validation
Conduct thorough testing to ensure that the AI models are accurately predicting outcomes and optimizing energy systems. Validate the results against known data to verify the effectiveness of the AI integration.
Deployment and Monitoring
Deploy the AI-enhanced solar system into production, closely monitoring its performance. Establish protocols for real-time monitoring and data analysis to identify any issues or improvements needed.
Troubleshooting Common Implementation Challenges
Despite careful planning, challenges may arise during the implementation of AI-enhanced solar systems. Here are common challenges and strategies for troubleshooting:
Common Issues | Solution |
---|---|
Poor-quality or insufficient data can hinder AI performance. | Implement data quality checks, invest in data cleaning processes, and ensure ongoing data integrity. |
Resistance from staff or stakeholders can impede the adoption of AI | Conduct change management initiatives, provide education on the benefits, and involve key stakeholders in the decision-making process. |
Inaccuracies in AI predictions may occur. | Continuously refine and update machine learning models based on real-world performance. Regularly validate and calibrate algorithms. |
Integrating AI with existing control systems may be complex. | Work closely with system integrators, ensure compatibility, and perform thorough testing before full deployment. |
Limited resources for implementation and maintenance. | Prioritize tasks, allocate resources efficiently, and explore partnerships or external support if necessary. |
Conclusion
AI is ushering in a new era of solar energy utilization, making it more accessible and efficient than ever before. With predictive analytics, efficiency enhancements, and remote monitoring, solar power is becoming a reliable and cost-effective energy source. As we embrace AI's potential, we move closer to a sustainable and renewable energy future, powered by the sun's boundless energy.
Other Related Resources
Here are several resources that provide in-depth insights into how AI is revolutionizing energy harvesting and optimization, along with brief summaries of each:
"Here's How AI Will Accelerate the Energy Transition" - World Economic Forum
This report discusses how AI can be a critical enabler for the energy transition. It outlines the necessary actions to unlock AI's potential in this domain, emphasizing its importance in driving a sustainable energy future.
This article explores the emergence of AI in the renewable energy sector and its transformative impact on energy harvesting and utilization. It highlights AI's contribution to creating a more sustainable future and its role in combating climate change.
The World Economic Forum's report, featured by Microsoft, emphasizes the significant role of AI in the energy transition. It provides an intriguing statistic that every 1 percent increase in efficiency in demand can create substantial economic value, thanks to reduced investment needs.
This resource discusses the future joint operation of energy harvesting and AI technology. It delves into the implementation of AI for data recognition, handling, and processing in energy harvesting systems, highlighting the use of optimization algorithms for finding favorable solutions.
This article on Nature.com addresses the critical global challenge of transitioning from fossil fuels to renewable energy sources. It underscores the need for advances in materials, devices, and systems for efficient energy harvesting, emphasizing the role of machine learning in this transition.
"Boosting Energy Harvesting via Deep Learning-Based Renewable Power Generation Prediction" - ScienceDirect
This piece of literature focuses on the improvements in power generation and consumption forecasting, particularly relevant to the operations of smart grids. It illustrates how deep learning-based approaches can enhance the balance of energy generation and consumption in connected systems.